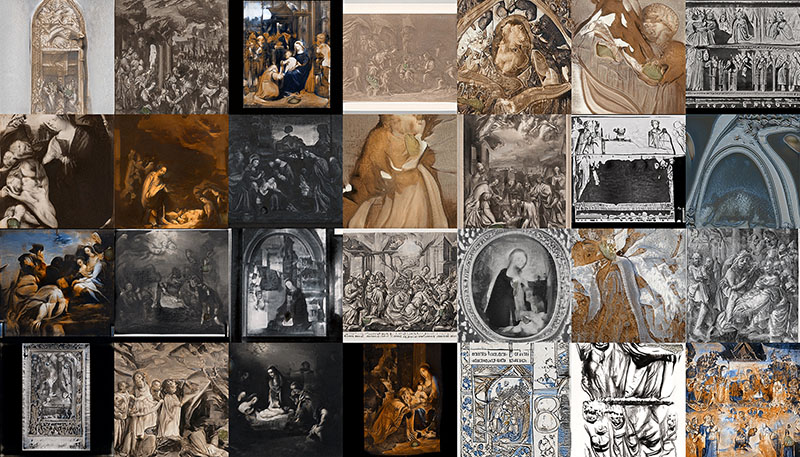
Synthetic “adorations”, produced by a generative adversarial network.
PROJECT ABSTRACT
Digital images enable us to virtually assemble, group, and rearrange works of art as image datasets. The highly complex similarities and dissimilarities between data points in an image dataset can be analyzed. Understanding the meaning of computationally defined similarities and dissimilarities, however, requires disentangling the representations learned by the computer in the process. By utilizing generative methods from deep learning, we aim to design a new methodology for the analysis and interpretation of digital images. Building upon refined methods of disentanglement from computer science, our goal is to establish the synthetic image as a novel means of knowledge production in art history.
TEAM
Peter Bell, Friedrich-Alexander-Universität Erlangen-Nürnberg (University of Erlangen-Nürnberg)
Björn Ommer, Ruprecht Karl University of Heidelberg
Fabian Offert, Friedrich-Alexander-Universität Erlangen-Nürnberg (University of Erlangen-Nürnberg)
Matthias Wright, Ruprecht Karl University of Heidelberg
LINK
https://www.kunstgeschichte.phil.fau.de/forschung/forschungsprojekte/bildsynthese/